The Problem
The financial services industry relies heavily on manual labor to reconcile and oversee millions of financial transactions per day. This is needed because older, disparate accounting systems often cannot keep pace with changes in regulations, terms, and pricing of newer financial products. To address these changes, financial services firms depend on human workers in order to review simple mathematical calculations, identify errors, and make adjustments. These workers often perform their tasks using spreadsheets, which generally offer no guidance on workers’ performance or accuracy.
Undetected mathematical errors can cost firms millions of dollars annually. For example, firms may need to compensate investors for mispricing trades or overcharging for fees. To prevent this, risk managers often require oversight processes where analysts are directed to double check calculations. But how effective are humans at identifying errors over time? Compounding this question is the method financial services firms employ to identify potential errors. Firms often apply thresholds to trigger alerts for review. These thresholds are commonly set at 1.5 to 2 standard deviations from an historic mean.
A Scientific Experiment
To analyze the effects of our interventions on participant performance, we used a negative binomial model of the number of errors that the subjects made over the course of 50 puzzles. All three treatments are included as indicator independent variables, and the control condition is omitted as a reference category. See Figures 1 and 2 below.
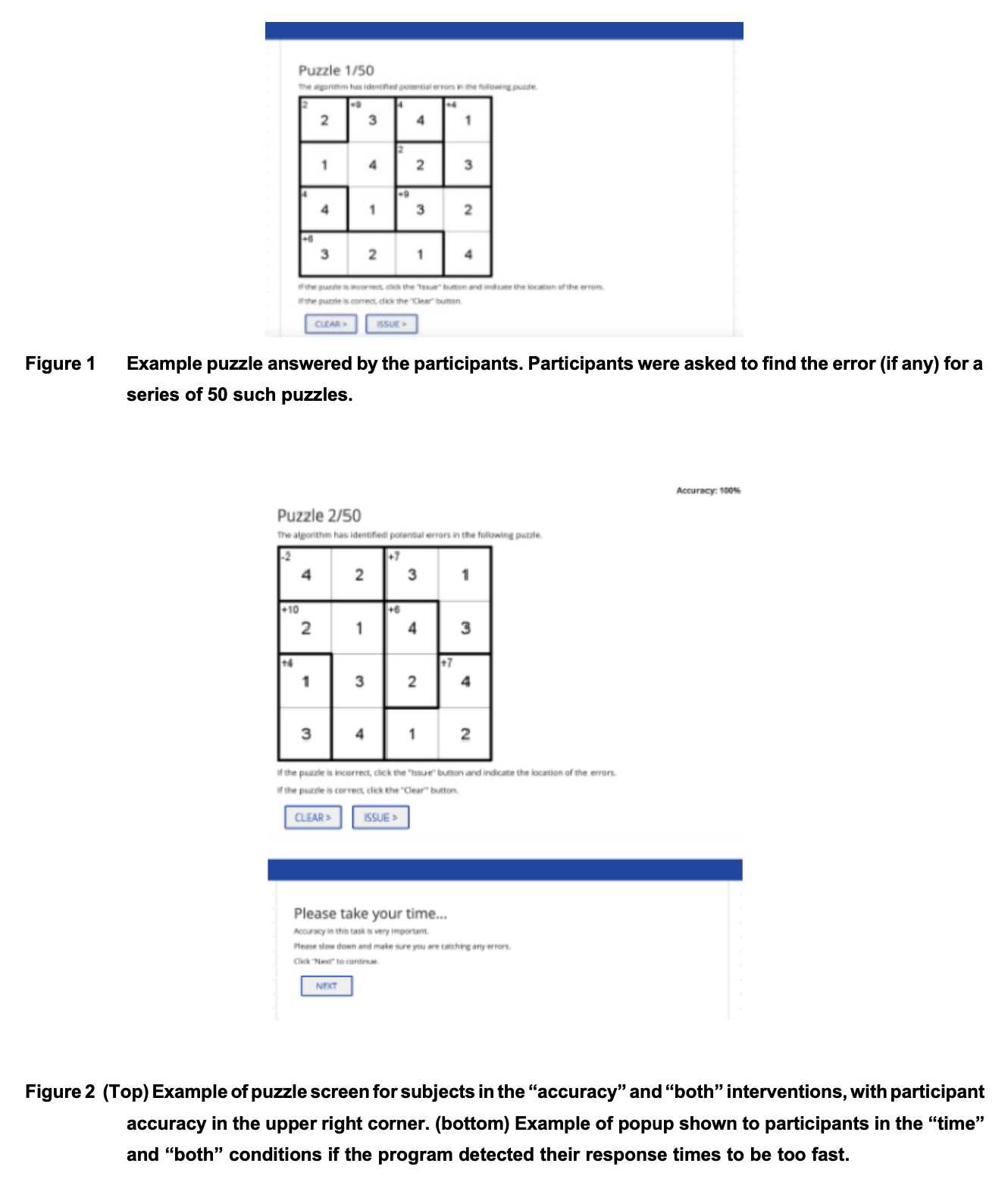
The Results
The table below reports the main result of our experiment as estimated marginal effects of a negative binomial model of the number of errors subjects made over 50 puzzles. Both of our individual treatments lowered the number of false negatives significantly. The time treatment lowered the likelihood of an additional false positive by 86.9% (SE: 27.8%) in comparison to control (p = 0.002). The accuracy treatment lowered the probability of an additional false positive by 91.5% (SE: 28.2%) in comparison to control (p = 0.001). None of the remaining average treatment effects we estimated were statistically significant.
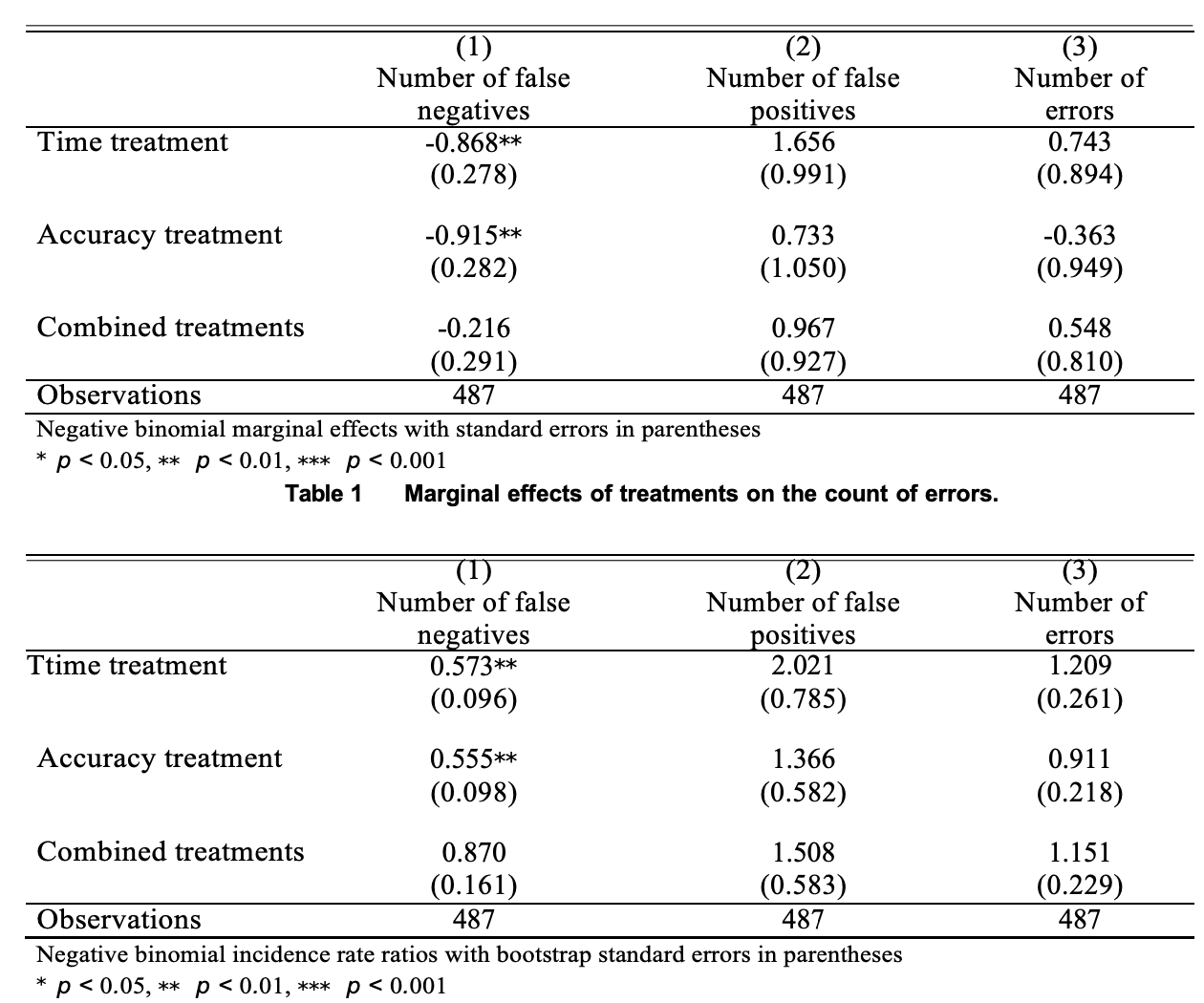