The Futility of Manual NAV Oversight
It is essential for every asset manager to provide independent oversight of their posted NAVs and reports. Even as asset managers outsource more of their accounting and administration to service providers, the one function they are directly responsible for is oversight. However, what does oversight mean and how far can it be taken with conventional methods? One of our asset manager clients processes the changes in over 2 million data points every day. It would take an army of staff to review each position. To address this paradox, the capital markets industry has taken oversight shortcuts. They review fund NAV changes that break a threshold, then try to find an explanation. Our work with Pimco has proven that AI systems can review each of the 2 million data points and learn to detect outliers. The algorithms can even search to find suspicious transactions that match similar errors. There is no doubt that AI oversight, combined with a smaller, expert team, is far superior to conventional oversight methods. In four years, we have identified over $100 million in errors missed by humans. This paper describes how we applied AI to beat the human oversight methods used by most firms.
How AI Oversight Works
Daily Data Ingestion. The first step in running an AI oversight method is reliable, daily data ingestion. As the diagram below indicates, our system ingests NAV data on a daily basis. We are quite experienced with ingesting data from the major banks. In particular, we have a platform and dedicated team at State Street who manage the OnCorps AI NAV system for Pimco and other firms. In most cases, we need only 2-3 weeks to set up a new firm’s data. In all cases, data are isolated and never integrated with other firms.
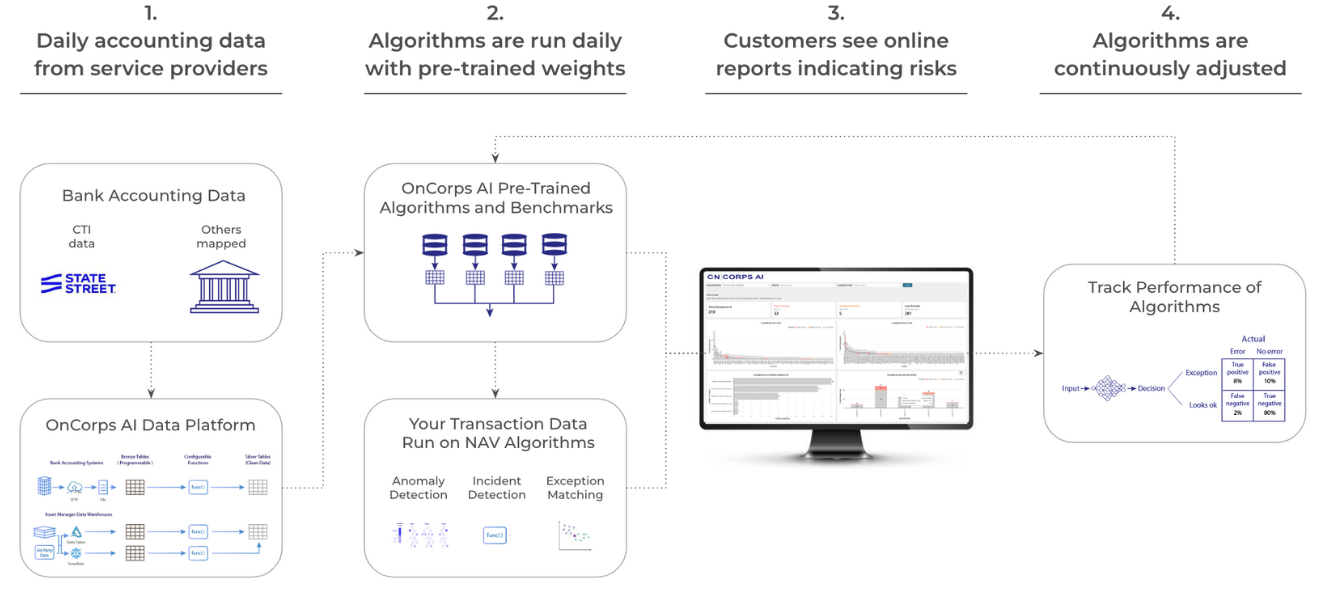
Pre-Trained Algorithms Track Individual Positions. As seen in step 2, data are processed by our pre-trained algorithms. We run anomaly detection, incident detection, and similarity scoring algorithms focused on income, capital pricing, and corporate actions. Working in combination, these algorithms help reduce statistical noise, pinpoint exceptions that may be real issues, and reduce redundant work on similar exceptions.
Online Dashboard of Risks. In step 3, we provide an online tool that tracks the high and medium risks by fund and CUSIP. Oversight professionals can see the results of the algorithm’s work and identify the incidents by basis point impact. This tool can also be used to prepare risk reports for meetings with service providers, auditors, and boards.
Performance Tracking and Continuous Fine-Tuning. Finally, we continuously track the algorithm’s performance against other firms in the system. To perform well, algorithms require continuous measurement and fine-tuning to perform well. This ensures we keep-up with changes in assets, transaction processing, and behaviors. Many internal and external AI teams are not setup to provide proper fine-tuning.
Reducing Exception Volume Without Missing Errors. As illustrated in the chart, our goal is to reduce oversight labor costs by reducing false positives and redundancies in exceptions. To do this, we apply three classes of algorithms. The first is anomaly detection. Here, we ask algorithms to find true outliers by running thousands of combinations of variables. This reduces false positives. The second are similarity scoring algorithms that match current exceptions with past resolved ones. This helps reduce time spent redundantly.
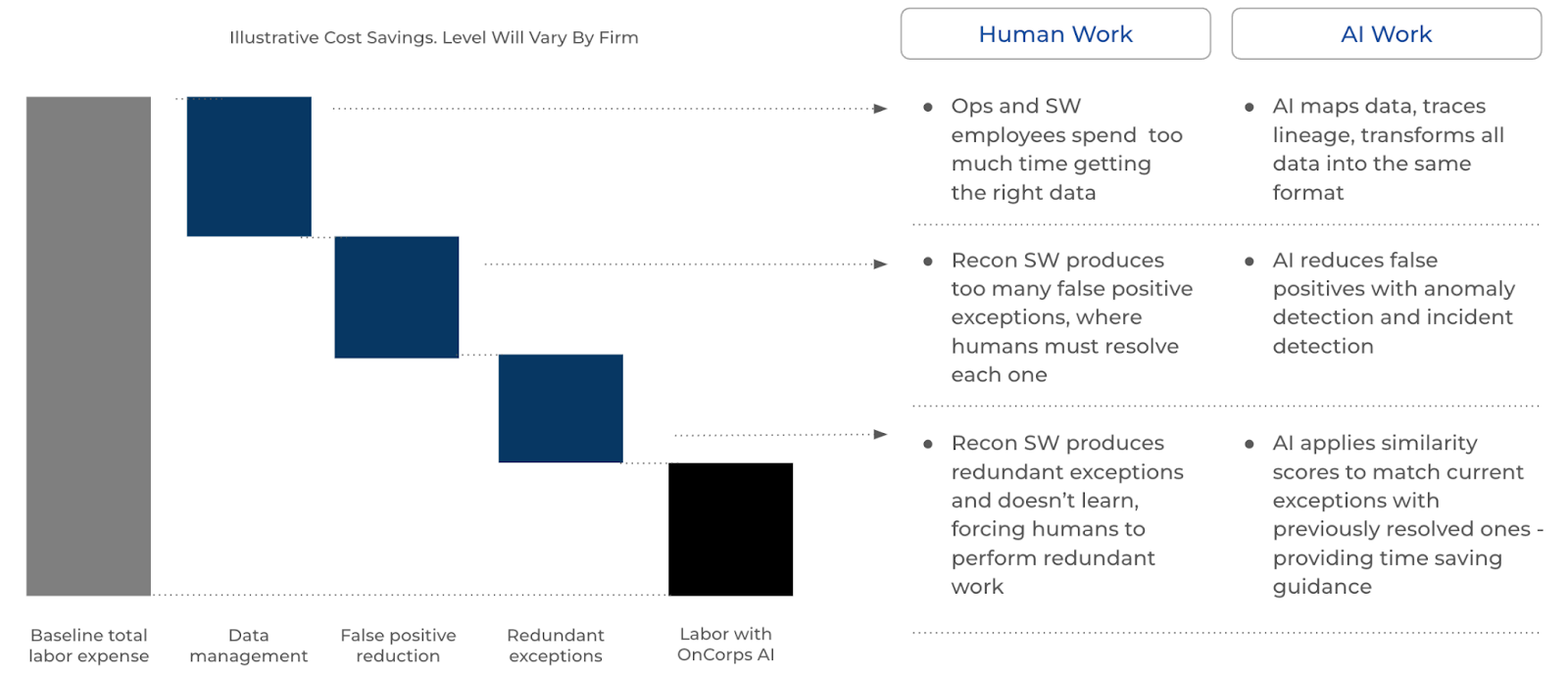
How AI Oversight Works
Incident Detection Algorithms. As we were fine-tuning the anomaly detection algorithm, we recognized the need to supplement the algorithm with specific functions to seek out and find indicators leading to past errors. We realized searching for these specific incidents also helped reduce the false positives. As new incidents arise, we have the ability to create, test and productionize new incident detection algorithms that can be shared across our network.
Current incident algorithms in production include;
- Abnormal Trade Size - Flags if an individual position has a daily income adjustment surpassing a materiality threshold.
- Amortization Shut Off on One Security - Flags if a position 'randomly' stops amortizing entirely on a date when it is not expected.
- Backdated Trades Check - Flags any BUY, SELL (for short and long positions) transaction where the contractual settle date does not equal the accounting date.
- Bad Cancel Rebook - Flags any item where there is a paydown that has been canceled and then rebooked.
- Bad Interest Sold - When a SELL transaction occurs, an issue is flagged if the interest bought / sold does not tie-out to expectation.
- Coupon Rate Check - Flags if the interest / coupon rate differs between positions of the same holding.
- Income Shut Off on One Security - Flags if a position 'randomly' stops accruing entirely on a date when it is not expected.
- Paydown Consistency - If more than one fund holds a position, then an exception is flagged if a paydown did not occur on one position, but occurred on others.
- Paydown Cancels - Flags any item where there is a paydown that has been canceled and then rebooked.
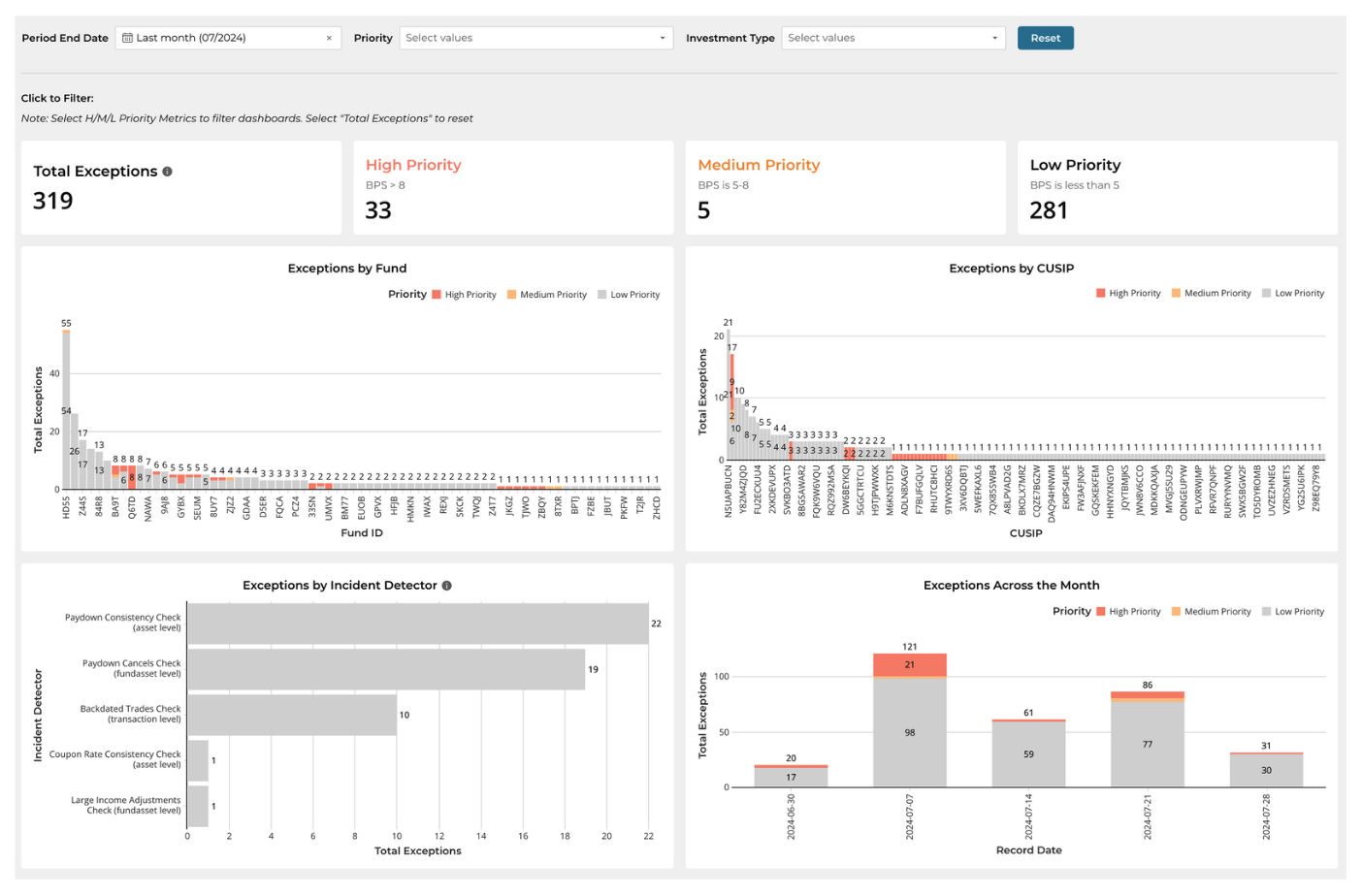
Online Summary of Anomalies and Exceptions. The NAV AI Oversight tool provides each customer a monthly online report of anomalies and exceptions by fund and CUSIP. These are ranked by frequency from left to right. They are also ranked by impact, red for high priority exceptions exceeding 8 basis points. Medium priority exceptions are estimated to have an impact of 5 to 8 basis points per fund. All of these parameters are adjustable.
Exciting Future Features. As we gain more data over time, key trend lines will be added to indicate whether risks are steady, declining or increasing. There are some quite unique features stemming from our shared algorithm model. We will reveal a benchmarking feature that highlights how your risks compare to others. Finally we will have the ability to send alerts to customers based on errors and trends that stem from common CUSIPS and incidents.
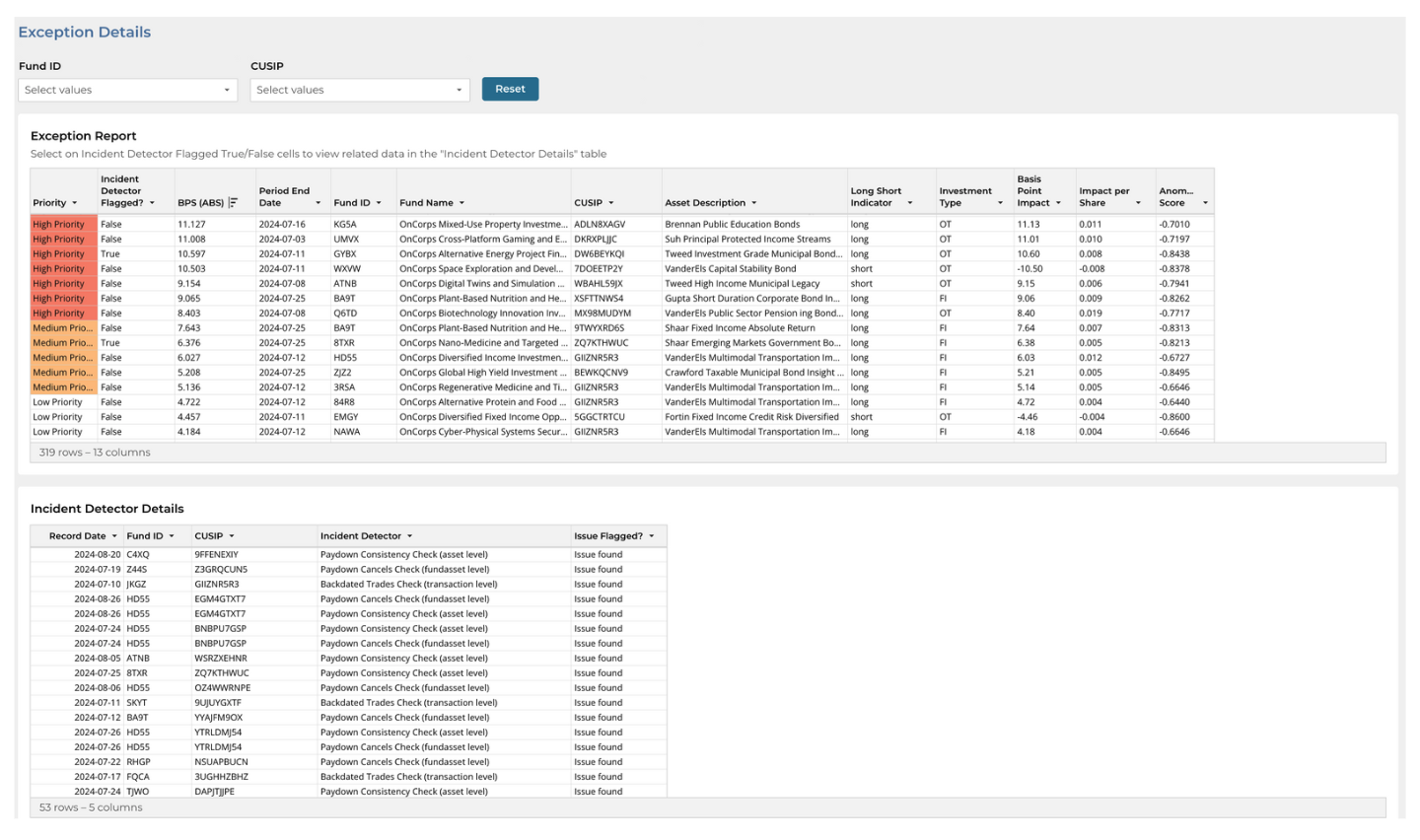
Click to See Exception Details. The standard dashboard allows users to see detailed exception lists by fund, CUSIP, and risk level. In the basic version of the system, users can download these monthly lists for discussions internally and with service providers. More sophisticated forms of our system enable the service provider to see the same online exceptions as their customers.
Sharing Algorithms in a Network
A Unique Shared Network Model. OnCorps AI has released a feature that enables us to share algorithms with all clients. This solution could have a profound impact on oversight. Algorithms that have been meticulously trained and fine-tuned over five years can now be shared with others. Specifically, common incidents can be alerted to others in the network. Today, most algorithms are self-contained within one firm’s systems. We realized that the problems our algorithms could solve would have common benefits. Because our algorithms were built to track individual positions, the unique security IDs such as CUSIPs would be searchable. One real life example is when a CUSIP for an asset backed security filed for bankruptcy, which was missed by an oversight team. Because the security was not treated as a bankrupt entity, it created a $1 million corporate action error. Incidents like these could likely trigger similar errors for firm’s holding the same securities (see the diagram below). As it indicates, the network model we developed would identify other firms holding the same CUSIP and alert them to a potential issue.
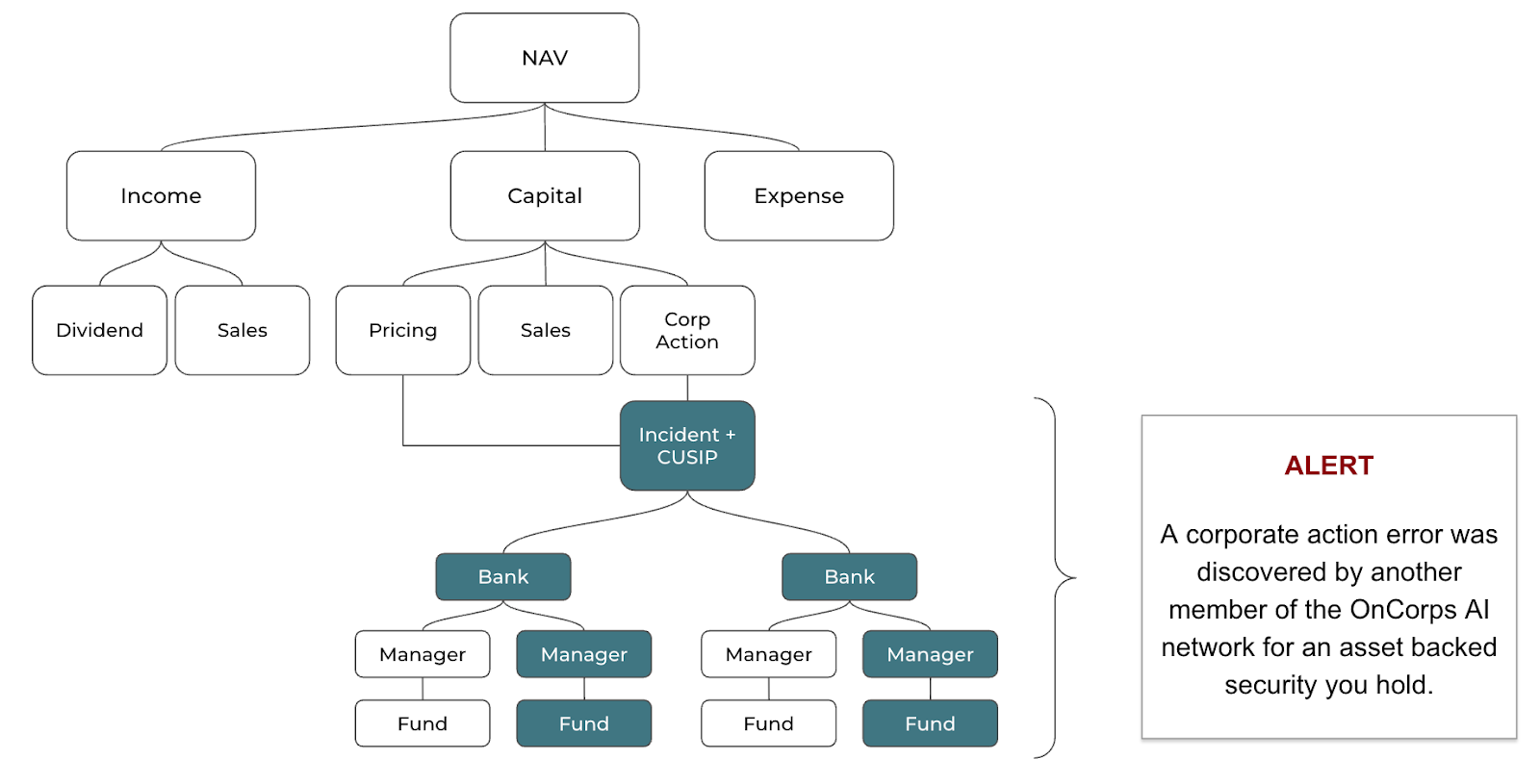
Benchmarking Service Provider Performance. Another major benefit of our shared algorithm platform is our ability to benchmark breaks as a percent of transactions. While this has many possible applications, the one that stands out is providing managers with a comparable assessment of service provider performance by asset class (see diagram below).

Results
$100 Million in Identified Errors. Over the past four years, we have identified over $100 million in NAV errors. We believe this record has been a result of the AI’s ability to read over 2 million rows a day while learning to seek errors.
Tested Accuracy Rates. Our algorithms have undergone detailed testing by major firms. Each exception flagged by our algorithms is verified by our customers on whether these are valid issues or false positives. Using this method, a recent test of our system resulted in a 94 percent accuracy rate. Moreover, when given a red-team test (a test with known planted errors) by a major bank, our algorithms identified over twice the number of issues, which were later deemed real issues by the bank.
Reduction in Exceptions. Our methods and algorithms systematically reduce exception volumes by as much as 90 percent. This is important because exception volumes correlate to labor rates.