OnCorps provides some of the largest asset managers in the world with production-grade AI to support investment operations and back office functions. We have learned quite a few lessons in training sophisticated algorithms to reduce time, labor, and error rates. I will focus on the first three lessons in this blog, illustrated below.

Labor Correlates to the Number of Exceptions
Compliance procedures require operations to track and resolve all exceptions produced by systems and documented procedures. Our experience is that most labor in financial and investment operations is spent chasing down exceptions. There is both a simple and statistical explanation for this. In the simplest terms, many large operations are experiencing a groundhog day effect, where they see the same types of exceptions over and over again. This is because the tools were not designed to learn and adjust their parameters. The statistical explanation is illustrated below. Because most operations require all transactions to be reviewed (illustrated in the large curve) and because true positives are rare (illustrated in the purple curve) a simple threshold break will produce mostly false positives.
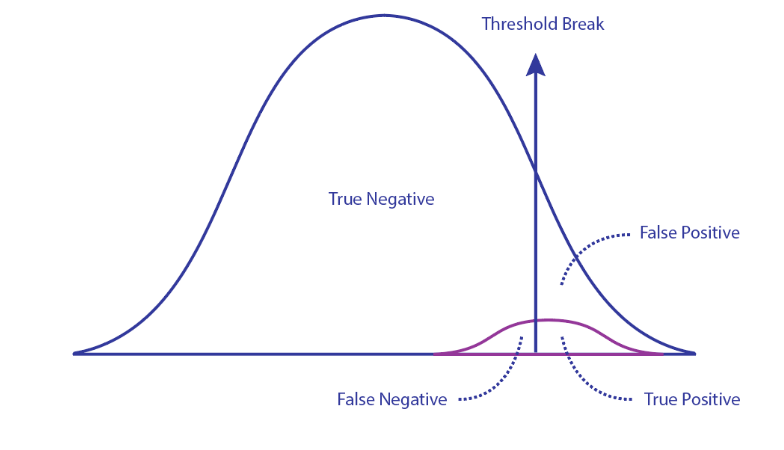
Even Advanced Recon Tools Generate Too Many Exceptions
It’s ironic that many firms spent millions on better reconciliation and workflow software only to find that labor rates persisted stubbornly. This is because these tools were designed to make it easy to configure. They therefore focused on the workflow more than the data. Our experience is that the data is by far the most important thing. Through constant algorithm fine tuning, we seek to produce data that minimizes false positives. Our goal is to get customers to spend upfront time confirming that our algorithms produce a 90% or greater set of exceptions that are deemed as worth seeing.
We have been quite successful applying anomaly detection algorithms, combined with incident detection checks, to reduce false positives. Combined, these algorithms have reduced the number of exceptions by over 90 percent, reducing the labor load by the same level (see illustration below). We are now testing algorithms that match past resolved exceptions with current exceptions. This lets analysts have more confidence in making faster decisions.

It’s Not a Reconciliation Problem, It’s an Explanation Problem
With over five years of fine tuning investment operations algorithms, we are convinced of the need for better root cause algorithms. In the past we have been diligent about a root cause “human in the loop” method that lets analysts click on the probable root cause. However, we have found that even this method has some flaws. Some transactions are rare and complex. On a busy day, it is easy to misattribute an exception. We are currently piloting a number of possible solutions. The first is a generative AI agent that helps explain the details of a transaction to make it easier for analysts to get the right context. The second is a time based algorithm that seeks to treat trades as if they were on a trip and predicts the delays based on known past factors.
Final Thoughts
It is easy to produce software and demos and hard to produce consistent outcomes. Training and fine tuning algorithms will not go away with generative AI. That is only one of a broad spectrum of algorithms needed to transform investment operations. Hopefully, others will learn from our lessons.